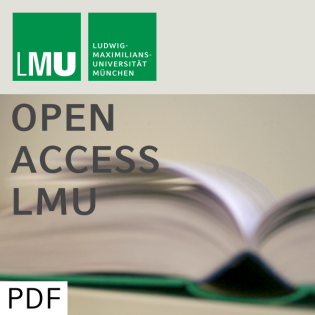
Maximum Likelihood Estimation in Graphical Models with Missing Values
Beschreibung
vor 27 Jahren
In this paper we discuss maximum likelihood estimation when some
observations are missing in mixed graphical interaction models
assuming a conditional Gaussian distribution as introduced by
Lauritzen&Wermuth (1989). For the saturated case ML estimation
with missing values via the EM algorithm has been proposed by
Little&Schluchter (1985). We expand their results to the
special restrictions in graphical models and indicate a more
efficient way to compute the E--step. The main purpose of the paper
is to show that for certain missing patterns the computational
effort can considerably be reduced.
observations are missing in mixed graphical interaction models
assuming a conditional Gaussian distribution as introduced by
Lauritzen&Wermuth (1989). For the saturated case ML estimation
with missing values via the EM algorithm has been proposed by
Little&Schluchter (1985). We expand their results to the
special restrictions in graphical models and indicate a more
efficient way to compute the E--step. The main purpose of the paper
is to show that for certain missing patterns the computational
effort can considerably be reduced.
Weitere Episoden


In Podcasts werben
Kommentare (0)