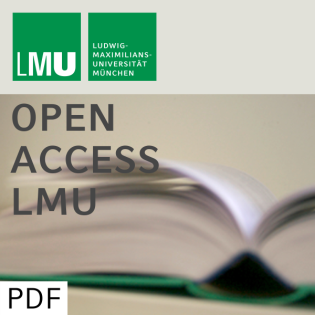
Dynamic and semiparametric models
Beschreibung
vor 27 Jahren
This paper surveys dynamic or state space models and their
relationship to non- and semiparametric models that are based on
the roughness penalty approach. We focus on recent advances in
dynamic modelling of non-Gaussian, in particular discrete-valued,
time series and longitudinal data, make the close correspondence to
semiparametric smoothing methods evident, and show how ideas from
dynamic models can be adopted for Bayesian semiparametric inference
in generalized additive and varying coefficient models. Basic tools
for corresponding inference techniques are penalized likelihood
estimation, Kalman filtering and smoothing and Markov chain Monte
Carlo (MCMC) simulation. Similarities, relative merits, advantages
and disadvantages of these methods are illustrated through several
applications.
relationship to non- and semiparametric models that are based on
the roughness penalty approach. We focus on recent advances in
dynamic modelling of non-Gaussian, in particular discrete-valued,
time series and longitudinal data, make the close correspondence to
semiparametric smoothing methods evident, and show how ideas from
dynamic models can be adopted for Bayesian semiparametric inference
in generalized additive and varying coefficient models. Basic tools
for corresponding inference techniques are penalized likelihood
estimation, Kalman filtering and smoothing and Markov chain Monte
Carlo (MCMC) simulation. Similarities, relative merits, advantages
and disadvantages of these methods are illustrated through several
applications.
Weitere Episoden


In Podcasts werben
Kommentare (0)