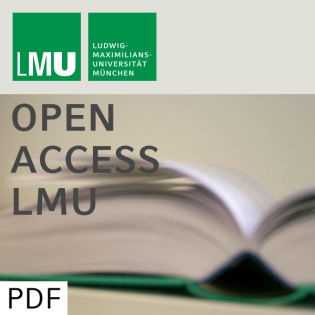
Average Run Length and Mean Delay for Changepoint Detection: Robust Estimates for Threshold Alarms
Beschreibung
vor 27 Jahren
Online Monitoring is a rapidly expanding field in different areas
such as quality control, finance and navigation. The automated
detection of so-called changepoints is playing a prominent role in
all these fields, be it the detection of sudden shifts of the mean
of a continuously monitored quantity, the variance of stock quotes
or the change of some characteristic features indicating the
malfunctioning of one of the detectors used for navigation (the
``faulty sensor problem''). A prominent example for the application
of advanced statistical methods for the detection of changepoints
in biomedical time series is the multi-process Kalman filter used
by Smith and West [Smith 1983] to monitor renal transplants.
However, despite the fact that the algorithm could be tuned in such
a way that the computer could predict dangerous situations on the
average one day before the human experts it has nevertheless become
superfluous as soon as new diagnosic tools became available. Many
of the automated monitoring systems which are widely used in
practice are based on simple threshold alarms. Some upper and lower
limits are chosen at the beginning of the monitoring session and an
alarm is triggered whenever the measured values exceed the upper
limit or fall below the lower limit. This is e.g. common practice
for the monitoring of patients during surgery, where such
thresholds are chosen for heart rate, blood pressure etc. by the
anaesthesist. The fate of the multi-process Kalman filter for
monitoring renal transplants teaches two lessons: first, there is
considerable power in statistical methods to improve conventional
biomedical monitoring techniques. Second, if the statistical model
and the methods are too refined they may never be used in practice.
We shall suggest a stochastic model for changepoints which we have
found to have the capacity to be very useful in practice, i.e.
which is sufficiently complex to cover the important features of a
changepoint system but simple enough to be understandable and
adaptible. We focus our attention on the properties of the
threshold alarm for different values of the parameters of the
threshold alarm and the model. This will give us practically
relevant estimates for this important class of alarm systems and
moreover a benchmark for the evaluation of competing alternative
algorithms. Note that virtually every algorithm designed to detect
changepoints is based on a threshold alarm, the only difference
being that the threshold alarm is not fed with the original data
but by a transformation thereof, usually called ``residuum''
[Basseville 1993]. As a general measure for quality, we look on the
one hand at the mean delay time $\tau$ between a changepoint and
its detection and on the other hand at the mean waiting time for a
false alarm, the so-called average run length ARL.
such as quality control, finance and navigation. The automated
detection of so-called changepoints is playing a prominent role in
all these fields, be it the detection of sudden shifts of the mean
of a continuously monitored quantity, the variance of stock quotes
or the change of some characteristic features indicating the
malfunctioning of one of the detectors used for navigation (the
``faulty sensor problem''). A prominent example for the application
of advanced statistical methods for the detection of changepoints
in biomedical time series is the multi-process Kalman filter used
by Smith and West [Smith 1983] to monitor renal transplants.
However, despite the fact that the algorithm could be tuned in such
a way that the computer could predict dangerous situations on the
average one day before the human experts it has nevertheless become
superfluous as soon as new diagnosic tools became available. Many
of the automated monitoring systems which are widely used in
practice are based on simple threshold alarms. Some upper and lower
limits are chosen at the beginning of the monitoring session and an
alarm is triggered whenever the measured values exceed the upper
limit or fall below the lower limit. This is e.g. common practice
for the monitoring of patients during surgery, where such
thresholds are chosen for heart rate, blood pressure etc. by the
anaesthesist. The fate of the multi-process Kalman filter for
monitoring renal transplants teaches two lessons: first, there is
considerable power in statistical methods to improve conventional
biomedical monitoring techniques. Second, if the statistical model
and the methods are too refined they may never be used in practice.
We shall suggest a stochastic model for changepoints which we have
found to have the capacity to be very useful in practice, i.e.
which is sufficiently complex to cover the important features of a
changepoint system but simple enough to be understandable and
adaptible. We focus our attention on the properties of the
threshold alarm for different values of the parameters of the
threshold alarm and the model. This will give us practically
relevant estimates for this important class of alarm systems and
moreover a benchmark for the evaluation of competing alternative
algorithms. Note that virtually every algorithm designed to detect
changepoints is based on a threshold alarm, the only difference
being that the threshold alarm is not fed with the original data
but by a transformation thereof, usually called ``residuum''
[Basseville 1993]. As a general measure for quality, we look on the
one hand at the mean delay time $\tau$ between a changepoint and
its detection and on the other hand at the mean waiting time for a
false alarm, the so-called average run length ARL.
Weitere Episoden


In Podcasts werben
Kommentare (0)