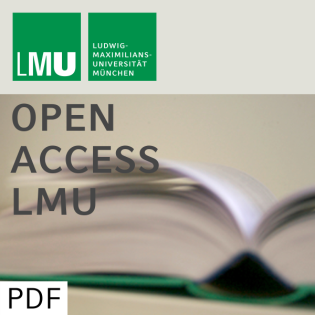
Penalized likelihood smoothing in robust state space models
Beschreibung
vor 26 Jahren
In likelihood-based approaches to robustify state space models,
Gaussian error distributions are replaced by non-normal
alternatives with heavier tails. Robustified observation models are
appropriate for time series with additive outliers, while state or
transition equations with heavy-tailed error distributions lead to
filters and smoothers that can cope with structural changes in
trend or slope caused by innovations outliers. As a consequence,
however, conditional filtering and smoothing densities become
analytically intractable. Various attempts have been made to deal
with this problem, reaching from approximate conditional mean type
estimation to fully Bayesian analysis using MCMC simulation. In
this article we consider penalized likelihood smoothers, this means
estimators which maximize penalized likelihoods or, equivalently,
posterior densities. Filtering and smoothing for additive and
innovations outlier models can be carried out by computationally
efficient Fisher scoring steps or iterative Kalman-type filters.
Special emphasis is on the Student family, for which EM-type
algorithms to estimate unknown hyperparameters are developed.
Operational behaviour is illustrated by simulation experiments and
by real data applications.
Gaussian error distributions are replaced by non-normal
alternatives with heavier tails. Robustified observation models are
appropriate for time series with additive outliers, while state or
transition equations with heavy-tailed error distributions lead to
filters and smoothers that can cope with structural changes in
trend or slope caused by innovations outliers. As a consequence,
however, conditional filtering and smoothing densities become
analytically intractable. Various attempts have been made to deal
with this problem, reaching from approximate conditional mean type
estimation to fully Bayesian analysis using MCMC simulation. In
this article we consider penalized likelihood smoothers, this means
estimators which maximize penalized likelihoods or, equivalently,
posterior densities. Filtering and smoothing for additive and
innovations outlier models can be carried out by computationally
efficient Fisher scoring steps or iterative Kalman-type filters.
Special emphasis is on the Student family, for which EM-type
algorithms to estimate unknown hyperparameters are developed.
Operational behaviour is illustrated by simulation experiments and
by real data applications.
Weitere Episoden


In Podcasts werben
Kommentare (0)