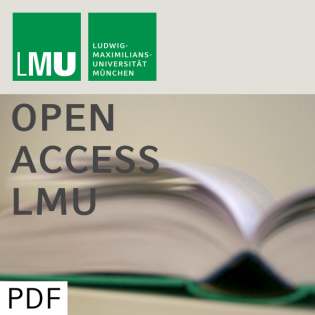
An Adaptive Gradient Algorithm for Maximum Likelihood Estimation in Imaging
Beschreibung
vor 26 Jahren
Markov random fields serve as natural models for patterns or
textures with random fluctuations at small scale. Given a general
form of such fields each class of pattern corresponds to a
collection of model parameters which critically determines the
abilitity of algorithms to segment or classify. Statistical
inference on parameters is based on (dependent) data given by a
portion of patterns inside some observation window. Unfortunately,
the corresponding maximum likelihood estimators are computationally
intractable by classical methods. Until recently, they even were
regarded as intractable at all. In recent years stochastic gradient
algorithms for their computation were proposed and studied. An
attractive class of such algorithms are those derived from adaptive
algorithms, wellknown in engeneering for a long time. We derive
convergence theorems following closely the lines proposed by M.
Metivier and P. Priouret (1987). This allows a transparent (albeit
somewhat technical) treatment. The results are weaker than those
obtained by L. Younes (1988). Keywords: adaptive algorithm,
stochastic approximation, stochastic gradient descent, MCMC
methods, maximum likelihood, Gibbs fields, imaging
textures with random fluctuations at small scale. Given a general
form of such fields each class of pattern corresponds to a
collection of model parameters which critically determines the
abilitity of algorithms to segment or classify. Statistical
inference on parameters is based on (dependent) data given by a
portion of patterns inside some observation window. Unfortunately,
the corresponding maximum likelihood estimators are computationally
intractable by classical methods. Until recently, they even were
regarded as intractable at all. In recent years stochastic gradient
algorithms for their computation were proposed and studied. An
attractive class of such algorithms are those derived from adaptive
algorithms, wellknown in engeneering for a long time. We derive
convergence theorems following closely the lines proposed by M.
Metivier and P. Priouret (1987). This allows a transparent (albeit
somewhat technical) treatment. The results are weaker than those
obtained by L. Younes (1988). Keywords: adaptive algorithm,
stochastic approximation, stochastic gradient descent, MCMC
methods, maximum likelihood, Gibbs fields, imaging
Weitere Episoden


In Podcasts werben
Kommentare (0)