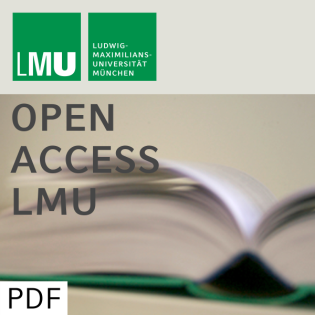
Solving Generalised Estimating Equations With Missing Data Using Pseudo Maximum Likelihood Estimation Is Equivalent to Complete Case Analysis
Beschreibung
vor 26 Jahren
Arminger and Sobel(1990) proposed an approach to estimate mean- and
covariance structures in the presence of missing data. These
authors claimed that their method based on Pseudo Maximum
Likelihood (PML) estimation may be applied if the data are missing
at random (MAR) in the sense of Little and Rubin (1987). Rotnitzky
and Robins (1995), however, stated that the PML approach may yield
inconsistent estimates if the data are (MAR). We show that the
adoption of the PML approach for mean- and covariance structures to
mean structures in the presence of missing data as proposed by
Ziegler (1994) is identical to the complete case (CC) estimator.
Nevertheless, the PML approach has the computational advantage in
that the association structure remains the same.
covariance structures in the presence of missing data. These
authors claimed that their method based on Pseudo Maximum
Likelihood (PML) estimation may be applied if the data are missing
at random (MAR) in the sense of Little and Rubin (1987). Rotnitzky
and Robins (1995), however, stated that the PML approach may yield
inconsistent estimates if the data are (MAR). We show that the
adoption of the PML approach for mean- and covariance structures to
mean structures in the presence of missing data as proposed by
Ziegler (1994) is identical to the complete case (CC) estimator.
Nevertheless, the PML approach has the computational advantage in
that the association structure remains the same.
Weitere Episoden


In Podcasts werben
Kommentare (0)