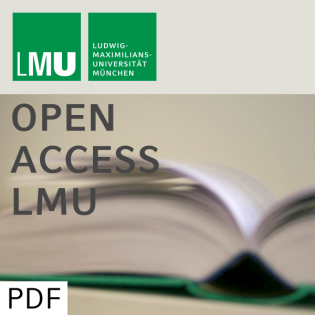
Bayesian Inference for Generalized Additive Regression based on Dynamic Models
Beschreibung
vor 26 Jahren
We present a general approach for Bayesian inference via Markov
chain Monte Carlo (MCMC) simulation in generalized additive,
semiparametric and mixed models. It is particularly appropriate for
discrete and other fundamentally non-Gaussian responses, where
Gibbs sampling techniques developed for Gaussian models cannot be
applied. We use the close relation between nonparametric regression
and dynamic or state space models to develop posterior sampling
procedures that are based on recent Metropolis-Hasting algorithms
for dynamic generalized linear models. We illustrate the approach
with applications to credit scoring and unemployment duration.
chain Monte Carlo (MCMC) simulation in generalized additive,
semiparametric and mixed models. It is particularly appropriate for
discrete and other fundamentally non-Gaussian responses, where
Gibbs sampling techniques developed for Gaussian models cannot be
applied. We use the close relation between nonparametric regression
and dynamic or state space models to develop posterior sampling
procedures that are based on recent Metropolis-Hasting algorithms
for dynamic generalized linear models. We illustrate the approach
with applications to credit scoring and unemployment duration.
Weitere Episoden


In Podcasts werben
Kommentare (0)