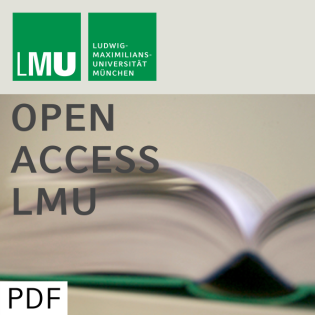
On Dependency in Double-Hurdle Models
Beschreibung
vor 26 Jahren
In microeconometrics, consumption data is typically zero-inflated
due to many individuals recording, for one reason or another, no
consumption. A mixture model can be appropriate for statistical
analysis of such data, with the Dependent Double-Hurdle model (DDH
hereafter) one specification that is frequently adopted in
econometric practice. Essentially, the DDH model is designed to
explain individual demand through a sequential two-step process: a
market participation decision (first hurdle), followed by a
consumption level decision (second hurdle) - a non-zero
correlation/covariance parameter allows for dependency between the
hurdles. A significant feature of the majority of empirical DDH
studies has been the lack of support for the existence of
dependency. This empirical phenomenon is studied from a theoretical
perspective using examples based on the bivariate normal, bivariate
logistic, and bivariate Poisson distributions. The Fisher
Information matrix for the parameters of the model is considered,
especially the component corresponding to the dependency parameter.
The main finding is that the DDH model contains too little
statistical information to support estimation of dependency, even
when dependency is truly present. Consequently, the paper calls for
the elimination of attempts to estimate dependency using the DDH
framework. The advantage of this strategy is that it permits
flexible modelling; some possibilities are proposed.
due to many individuals recording, for one reason or another, no
consumption. A mixture model can be appropriate for statistical
analysis of such data, with the Dependent Double-Hurdle model (DDH
hereafter) one specification that is frequently adopted in
econometric practice. Essentially, the DDH model is designed to
explain individual demand through a sequential two-step process: a
market participation decision (first hurdle), followed by a
consumption level decision (second hurdle) - a non-zero
correlation/covariance parameter allows for dependency between the
hurdles. A significant feature of the majority of empirical DDH
studies has been the lack of support for the existence of
dependency. This empirical phenomenon is studied from a theoretical
perspective using examples based on the bivariate normal, bivariate
logistic, and bivariate Poisson distributions. The Fisher
Information matrix for the parameters of the model is considered,
especially the component corresponding to the dependency parameter.
The main finding is that the DDH model contains too little
statistical information to support estimation of dependency, even
when dependency is truly present. Consequently, the paper calls for
the elimination of attempts to estimate dependency using the DDH
framework. The advantage of this strategy is that it permits
flexible modelling; some possibilities are proposed.
Weitere Episoden


In Podcasts werben
Kommentare (0)