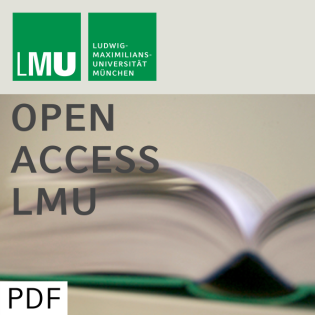
Bayesian analysis of logistic regression with an unknown change point
Beschreibung
vor 25 Jahren
We discuss Bayesian estimation of a logistic regression model with
an unknown threshold limiting value (TLV). In these models it is
assumed that there is no effect of a covariate on the response
under a certain unknown TLV. The estimation of these models with a
focus on the TLV in a Bayesian context by Markov chain Monte Carlo
(MCMC) methods is considered. We extend the model by accounting for
measurement error in the covariate. The Bayesian solution is
compared with the likelihood solution proposed by Kuechenhoff and
Carroll (1997) using a data set concerning the relationship between
dust concentration in the working place and the occurrence of
chronic bronchitis.
an unknown threshold limiting value (TLV). In these models it is
assumed that there is no effect of a covariate on the response
under a certain unknown TLV. The estimation of these models with a
focus on the TLV in a Bayesian context by Markov chain Monte Carlo
(MCMC) methods is considered. We extend the model by accounting for
measurement error in the covariate. The Bayesian solution is
compared with the likelihood solution proposed by Kuechenhoff and
Carroll (1997) using a data set concerning the relationship between
dust concentration in the working place and the occurrence of
chronic bronchitis.
Weitere Episoden


In Podcasts werben
Kommentare (0)