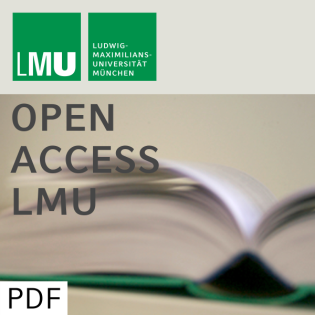
Inference in High Dimensional Generalized Linear Models based on Soft-Thresholding
Beschreibung
vor 25 Jahren
We propose a new method for estimation of a high number of
coefficients within the generalized linear model framework. The
estimator leads to an adaptive selection of model terms without
substantial variance inflation. Our proposal extends the
soft-thresholding strategy from Donoho and Johnstone (1994) to
generalized linear models and multiple predictor variables.
Furthermore, we develop an estimator for the covariance matrix of
the estimated coefficients, which can even be used for terms
dropped from the model. Used in connection with basis functions,
the proposed methodology provides an alternative to other
generalized function estimators. It leads to an adaptive economical
description of the results in terms of basis functions.
Specifically, it is shown how adaptive regression splines and
qualitative restrictions can be incorporated. Our approach is
demonstrated by applications to solvency prognosis and rental
guides.
coefficients within the generalized linear model framework. The
estimator leads to an adaptive selection of model terms without
substantial variance inflation. Our proposal extends the
soft-thresholding strategy from Donoho and Johnstone (1994) to
generalized linear models and multiple predictor variables.
Furthermore, we develop an estimator for the covariance matrix of
the estimated coefficients, which can even be used for terms
dropped from the model. Used in connection with basis functions,
the proposed methodology provides an alternative to other
generalized function estimators. It leads to an adaptive economical
description of the results in terms of basis functions.
Specifically, it is shown how adaptive regression splines and
qualitative restrictions can be incorporated. Our approach is
demonstrated by applications to solvency prognosis and rental
guides.
Weitere Episoden


In Podcasts werben
Kommentare (0)