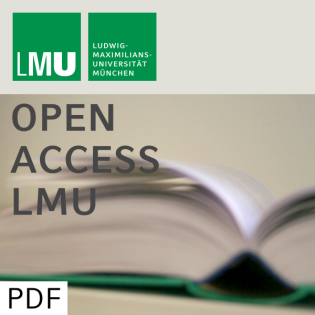
Marginal Regression Models with Varying Coefficients for Correlated Ordinal Data
Beschreibung
vor 25 Jahren
This paper discusses marginal regression models for repeated or
clustered ordinal measurements in which the coefficients of
explanatory variables are allowed to vary as smooth functions of
other covariates. We model the marginal response probabilities and
the marginal pairwise association structure by two semiparametric
regressions. To estimate the fixed parameters and varying
coefficients in both models we derive an algorithm that is based on
penalized generalized estimating equations. This allows to estimate
the marginal model without specifying the entire distribution of
the correlated categorical response variables. Our implementation
of the estimation algorithm uses an orthonormal cubic spline basis
that separates the estimated varying coefficients into a linear
part and a smooth curvature part. By avoiding an additional
backfitting step in the optimization procedure we are able to
compute a robust approximation for the covariance matrix of the
final estimate. We illustrate our method by an application to
longitudinal data from a forest damage survey. We show how to model
the dependence of damage state of beeches on non-linear trend
functions and time-varying effects of age.
clustered ordinal measurements in which the coefficients of
explanatory variables are allowed to vary as smooth functions of
other covariates. We model the marginal response probabilities and
the marginal pairwise association structure by two semiparametric
regressions. To estimate the fixed parameters and varying
coefficients in both models we derive an algorithm that is based on
penalized generalized estimating equations. This allows to estimate
the marginal model without specifying the entire distribution of
the correlated categorical response variables. Our implementation
of the estimation algorithm uses an orthonormal cubic spline basis
that separates the estimated varying coefficients into a linear
part and a smooth curvature part. By avoiding an additional
backfitting step in the optimization procedure we are able to
compute a robust approximation for the covariance matrix of the
final estimate. We illustrate our method by an application to
longitudinal data from a forest damage survey. We show how to model
the dependence of damage state of beeches on non-linear trend
functions and time-varying effects of age.
Weitere Episoden


In Podcasts werben
Kommentare (0)