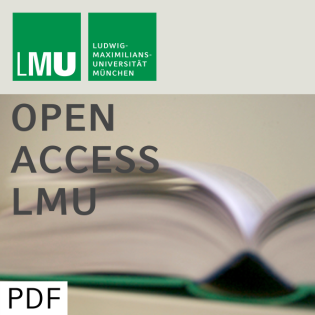
Bayesian generalized additive mixed models. A simulation study
Beschreibung
vor 23 Jahren
Generalized additive mixed models extend the common parametric
predictor of generalized linear models by adding unknown smooth
functions of different types of covariates as well as random
effects. From a Bayesian viewpoint, all effects as well as
smoothing parameters are random. Assigning appropriate priors,
posterior inference can be based on Markov chain Monte Carlo
techniques within a unified framework. Given observations on the
response and on covariates, questions like the following arise: Can
the additive structure be recovered? How well are unknown functions
and effects estimated? Is it possible to discriminate between
different types of random effects? The aim of this paper is to
obtain some answers to such questions through a careful simulation
study. Thereby, we focus on models for Gaussian and categorical
responses based on smoothness priors as in Fahrmeir and Lang
(2001). The result of the study provides valuable insight into the
facilities and limitations of the models when applying them to real
data.
predictor of generalized linear models by adding unknown smooth
functions of different types of covariates as well as random
effects. From a Bayesian viewpoint, all effects as well as
smoothing parameters are random. Assigning appropriate priors,
posterior inference can be based on Markov chain Monte Carlo
techniques within a unified framework. Given observations on the
response and on covariates, questions like the following arise: Can
the additive structure be recovered? How well are unknown functions
and effects estimated? Is it possible to discriminate between
different types of random effects? The aim of this paper is to
obtain some answers to such questions through a careful simulation
study. Thereby, we focus on models for Gaussian and categorical
responses based on smoothness priors as in Fahrmeir and Lang
(2001). The result of the study provides valuable insight into the
facilities and limitations of the models when applying them to real
data.
Weitere Episoden


In Podcasts werben
Kommentare (0)