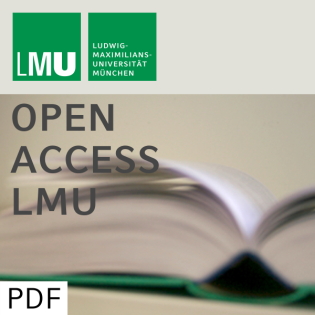
Testing for Reduction to Random Walk in Autoregressive Conditional Heteroskedasticity Models
Beschreibung
vor 23 Jahren
The AR-ARCH and AR-GARCH models, which allow for conditional
heteroskedasticity and autoregression, reduce to random walk or
white noise for some values of the parameters. We consider
generalised versions of the AR-ARCH(1) and AR-GARCH(1,1) models,
and, under mild assumptions, calculate the asymptotic distributions
of pseudo-likelihood ratio statistics for testing hypotheses that
reect these reductions. These hypotheses are of two kinds: the
conditional volatility parameters may take their boundary values of
zero, or the autoregressive component may take the form of a unit
root process or not in fact be present. The limiting distributions
of the resulting test statistics can be expressed in terms of
functionals of Brownian motion related to the Dickey-Fuller
statistic, together with independent chi-square components. The
finite sample performances of the test statistics are assessed by
simulations, and percentiles are tabulated. The results have
applications in the analysis of financial time series and random
coefficient models.
heteroskedasticity and autoregression, reduce to random walk or
white noise for some values of the parameters. We consider
generalised versions of the AR-ARCH(1) and AR-GARCH(1,1) models,
and, under mild assumptions, calculate the asymptotic distributions
of pseudo-likelihood ratio statistics for testing hypotheses that
reect these reductions. These hypotheses are of two kinds: the
conditional volatility parameters may take their boundary values of
zero, or the autoregressive component may take the form of a unit
root process or not in fact be present. The limiting distributions
of the resulting test statistics can be expressed in terms of
functionals of Brownian motion related to the Dickey-Fuller
statistic, together with independent chi-square components. The
finite sample performances of the test statistics are assessed by
simulations, and percentiles are tabulated. The results have
applications in the analysis of financial time series and random
coefficient models.
Weitere Episoden


In Podcasts werben
Kommentare (0)