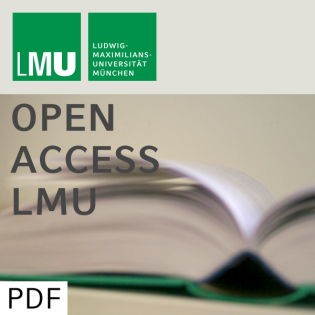
Bayesian varying-coefficient models using adaptive regression splines
Beschreibung
vor 23 Jahren
Varying-coefficient models provide a flexible framework for semi-
and nonparametric generalized regression analysis. We present a
fully Bayesian B-spline basis function approach with adaptive knot
selection. For each of the unknown regression functions or varying
coefficients, the number and location of knots and the B-spline
coefficients are estimated simultaneously using reversible jump
Markov chain Monte Carlo sampling. The overall procedure can
therefore be viewed as a kind of Bayesian model averaging. Although
Gaussian responses are covered by the general framework, the method
is particularly useful for fundamentally non-Gaussian responses,
where less alternatives are available. We illustrate the approach
with a thorough application to two data sets analysed previously in
the literature: the kyphosis data set with a binary response and
survival data from the Veteran’s Administration lung cancer trial.
and nonparametric generalized regression analysis. We present a
fully Bayesian B-spline basis function approach with adaptive knot
selection. For each of the unknown regression functions or varying
coefficients, the number and location of knots and the B-spline
coefficients are estimated simultaneously using reversible jump
Markov chain Monte Carlo sampling. The overall procedure can
therefore be viewed as a kind of Bayesian model averaging. Although
Gaussian responses are covered by the general framework, the method
is particularly useful for fundamentally non-Gaussian responses,
where less alternatives are available. We illustrate the approach
with a thorough application to two data sets analysed previously in
the literature: the kyphosis data set with a binary response and
survival data from the Veteran’s Administration lung cancer trial.
Weitere Episoden


In Podcasts werben
Kommentare (0)