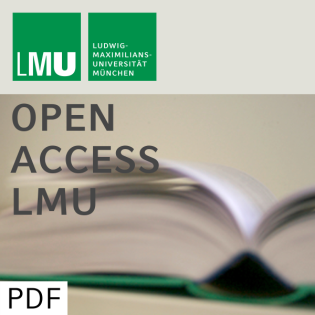
fMRI activation detection with EEG priors
Beschreibung
vor 11 Jahren
The purpose of brain mapping techniques is to advance the
understanding of the relationship between structure and function in
the human brain in so-called activation studies. In this work, an
advanced statistical model for combining functional magnetic
resonance imaging (fMRI) and electroencephalography (EEG)
recordings is developed to fuse complementary information about the
location of neuronal activity. More precisely, a new Bayesian
method is proposed for enhancing fMRI activation detection by the
use of EEG-based spatial prior information in stimulus based
experimental paradigms. I.e., we model and analyse stimulus
influence by a spatial Bayesian variable selection scheme, and
extend existing high-dimensional regression methods by
incorporating prior information on binary selection indicators via
a latent probit regression with either a spatially-varying or
constant EEG effect. Spatially-varying effects are regularized by
intrinsic Markov random field priors. Inference is based on a full
Bayesian Markov Chain Monte Carlo (MCMC) approach. Whether the
proposed algorithm is able to increase the sensitivity of mere fMRI
models is examined in both a real-world application and a
simulation study. We observed, that carefully selected EEG--prior
information additionally increases sensitivity in activation
regions that have been distorted by a low signal-to-noise ratio.
understanding of the relationship between structure and function in
the human brain in so-called activation studies. In this work, an
advanced statistical model for combining functional magnetic
resonance imaging (fMRI) and electroencephalography (EEG)
recordings is developed to fuse complementary information about the
location of neuronal activity. More precisely, a new Bayesian
method is proposed for enhancing fMRI activation detection by the
use of EEG-based spatial prior information in stimulus based
experimental paradigms. I.e., we model and analyse stimulus
influence by a spatial Bayesian variable selection scheme, and
extend existing high-dimensional regression methods by
incorporating prior information on binary selection indicators via
a latent probit regression with either a spatially-varying or
constant EEG effect. Spatially-varying effects are regularized by
intrinsic Markov random field priors. Inference is based on a full
Bayesian Markov Chain Monte Carlo (MCMC) approach. Whether the
proposed algorithm is able to increase the sensitivity of mere fMRI
models is examined in both a real-world application and a
simulation study. We observed, that carefully selected EEG--prior
information additionally increases sensitivity in activation
regions that have been distorted by a low signal-to-noise ratio.
Weitere Episoden
In Podcasts werben
Kommentare (0)