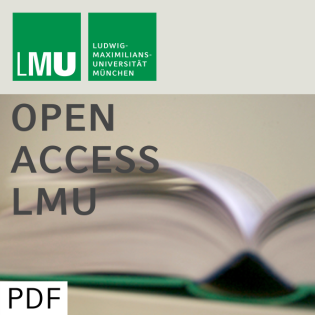
Tree-Structured Modelling of Categorical Predictors in Regression
Beschreibung
vor 10 Jahren
Generalized linear and additive models are very efficient
regression tools but the selection of relevant terms becomes
difficult if higher order interactions are needed. In contrast,
tree-based methods also known as recursive partitioning are
explicitly designed to model a specific form of interaction but
with their focus on interaction tend to neglect the main effects.
The method proposed here focusses on the main effects of
categorical predictors by using tree type methods to obtain
clusters. In particular when the predictor has many categories one
wants to know which of the categories have to be distinguished with
respect to their effect on the response. The tree-structured
approach allows to detect clusters of categories that share the
same effect while letting other variables, in particular metric
variables, have a linear or additive effect on the response. An
algorithm for the fitting is proposed and various stopping criteria
are evaluated. The preferred stopping criterion is based on
p-values representing a conditional inference procedure. In
addition, stability of clusters are investigated and the relevance
of variables is investigated by bootstrap methods. Several
applications show the usefulness of tree-structured clustering and
a small simulation study demonstrates that the fitting procedure
works well.
regression tools but the selection of relevant terms becomes
difficult if higher order interactions are needed. In contrast,
tree-based methods also known as recursive partitioning are
explicitly designed to model a specific form of interaction but
with their focus on interaction tend to neglect the main effects.
The method proposed here focusses on the main effects of
categorical predictors by using tree type methods to obtain
clusters. In particular when the predictor has many categories one
wants to know which of the categories have to be distinguished with
respect to their effect on the response. The tree-structured
approach allows to detect clusters of categories that share the
same effect while letting other variables, in particular metric
variables, have a linear or additive effect on the response. An
algorithm for the fitting is proposed and various stopping criteria
are evaluated. The preferred stopping criterion is based on
p-values representing a conditional inference procedure. In
addition, stability of clusters are investigated and the relevance
of variables is investigated by bootstrap methods. Several
applications show the usefulness of tree-structured clustering and
a small simulation study demonstrates that the fitting procedure
works well.
Weitere Episoden
In Podcasts werben
Kommentare (0)