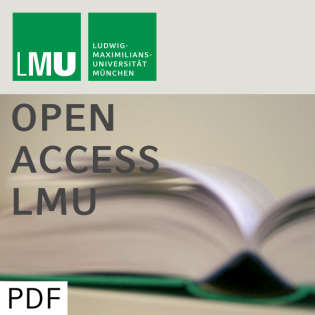
A variance decomposition and a Central Limit Theorem for empirical losses associated with resampling designs
Beschreibung
vor 10 Jahren
The mean prediction error of a classification or regression
procedure can be estimated using resampling designs such as the
cross-validation design. We decompose the variance of such an
estimator associated with an arbitrary resampling procedure into a
small linear combination of covariances between elementary
estimators, each of which is a regular parameter as described in
the theory of $U$-statistics. The enumerative combinatorics of the
occurrence frequencies of these covariances govern the linear
combination's coefficients and, therefore, the variance's large
scale behavior. We study the variance of incomplete U-statistics
associated with kernels which are partly but not entirely
symmetric. This leads to asymptotic statements for the prediction
error's estimator, under general non-empirical conditions on the
resampling design. In particular, we show that the resampling based
estimator of the average prediction error is asymptotically
normally distributed under a general and easily verifiable
condition. Likewise, we give a sufficient criterion for
consistency. We thus develop a new approach to understanding
small-variance designs as they have recently appeared in the
literature. We exhibit the $U$-statistics which estimate these
variances. We present a case from linear regression where the
covariances between the elementary estimators can be computed
analytically. We illustrate our theory by computing estimators of
the studied quantities in an artificial data example.
procedure can be estimated using resampling designs such as the
cross-validation design. We decompose the variance of such an
estimator associated with an arbitrary resampling procedure into a
small linear combination of covariances between elementary
estimators, each of which is a regular parameter as described in
the theory of $U$-statistics. The enumerative combinatorics of the
occurrence frequencies of these covariances govern the linear
combination's coefficients and, therefore, the variance's large
scale behavior. We study the variance of incomplete U-statistics
associated with kernels which are partly but not entirely
symmetric. This leads to asymptotic statements for the prediction
error's estimator, under general non-empirical conditions on the
resampling design. In particular, we show that the resampling based
estimator of the average prediction error is asymptotically
normally distributed under a general and easily verifiable
condition. Likewise, we give a sufficient criterion for
consistency. We thus develop a new approach to understanding
small-variance designs as they have recently appeared in the
literature. We exhibit the $U$-statistics which estimate these
variances. We present a case from linear regression where the
covariances between the elementary estimators can be computed
analytically. We illustrate our theory by computing estimators of
the studied quantities in an artificial data example.
Weitere Episoden
In Podcasts werben
Kommentare (0)