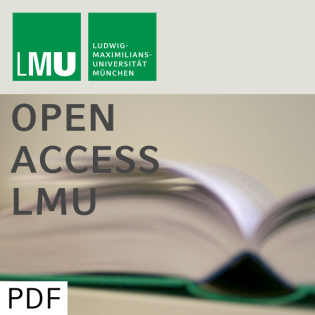
Estimating individual treatment effects from responses and a predictive biomarker in a parallel group RCT
Beschreibung
vor 9 Jahren
When being interested in administering the best of two treatments
to an individual patient i, it is necessary to know the individual
treatment effects (ITEs) of the considered subjects and the
correlation between the possible responses (PRs) for two
treatments. When data are generated in a parallel–group design RCT,
it is not possible to determine the ITE for a single subject since
we only observe two samples from the marginal distributions of
these PRs and not the corresponding joint distribution due to the
’Fundamental Problem of Causal Inference’ [Holland, 1986, p. 947].
In this article, we present a counterfactual approach for
estimating the joint distribution of two normally distributed
responses to two treatments. This joint distribution can be
estimated by assuming a normal joint distribution for the PRs and
by using a normally distributed baseline biomarker which is defined
to be functionally related to the sum of the ITE components. Such a
functional relationship is plausible since a biomarker and the sum
encode for the same information in a RCT, namely the variation
between subjects. As a result of the interpretation of the
biomarker as a proxy for the sum of ITE components, the estimation
of the joint distribution is subjected to some constraints. These
constraints can be framed in the context of linear regressions with
regard to the proportions of variances in the responses explained
and with regard to the residual variation. As a consequence, a new
light is thrown on the presence of treatment–biomarker
interactions. We applied our approach to a classical medical data
example on exercise and heart rate.
to an individual patient i, it is necessary to know the individual
treatment effects (ITEs) of the considered subjects and the
correlation between the possible responses (PRs) for two
treatments. When data are generated in a parallel–group design RCT,
it is not possible to determine the ITE for a single subject since
we only observe two samples from the marginal distributions of
these PRs and not the corresponding joint distribution due to the
’Fundamental Problem of Causal Inference’ [Holland, 1986, p. 947].
In this article, we present a counterfactual approach for
estimating the joint distribution of two normally distributed
responses to two treatments. This joint distribution can be
estimated by assuming a normal joint distribution for the PRs and
by using a normally distributed baseline biomarker which is defined
to be functionally related to the sum of the ITE components. Such a
functional relationship is plausible since a biomarker and the sum
encode for the same information in a RCT, namely the variation
between subjects. As a result of the interpretation of the
biomarker as a proxy for the sum of ITE components, the estimation
of the joint distribution is subjected to some constraints. These
constraints can be framed in the context of linear regressions with
regard to the proportions of variances in the responses explained
and with regard to the residual variation. As a consequence, a new
light is thrown on the presence of treatment–biomarker
interactions. We applied our approach to a classical medical data
example on exercise and heart rate.
Weitere Episoden
In Podcasts werben
Kommentare (0)