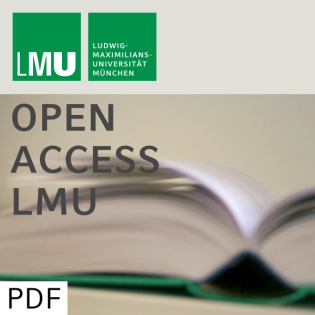
A comparison of different linkage statistics in small to moderate sized pedigrees with complex diseases
Podcast
Podcaster
Beschreibung
vor 12 Jahren
Background In the last years GWA studies have successfully
identified common SNPs associated with complex diseases. However,
most of the variants found this way account for only a small
portion of the trait variance. This fact leads researchers to focus
on rare-variant mapping with large scale sequencing, which can be
facilitated by using linkage information. The question arises why
linkage analysis often fails to identify genes when analyzing
complex diseases. Using simulations we have investigated the power
of parametric and nonparametric linkage statistics (KC-LOD, NPL,
LOD and MOD scores), to detect the effect of genes responsible for
complex diseases using different pedigree structures. Results As
expected, a small number of pedigrees with less than three affected
individuals has low power to map disease genes with modest effect.
Interestingly, the power decreases when unaffected individuals are
included in the analysis, irrespective of the true mode of
inheritance. Furthermore, we found that the best performing
statistic depends not only on the type of pedigrees but also on the
true mode of inheritance. Conclusions When applied in a sensible
way linkage is an appropriate and robust technique to map genes for
complex disease. Unlike association analysis, linkage analysis is
not hampered by allelic heterogeneity. So, why does linkage
analysis often fail with complex diseases? Evidently, when using an
insufficient number of small pedigrees, one might miss a true
genetic linkage when actually a real effect exists. Furthermore, we
show that the test statistic has an important effect on the power
to detect linkage as well. Therefore, a linkage analysis might fail
if an inadequate test statistic is employed. We provide
recommendations regarding the most favorable test statistics, in
terms of power, for a given mode of inheritance and type of
pedigrees under study, in order to reduce the probability to miss a
true linkage.
identified common SNPs associated with complex diseases. However,
most of the variants found this way account for only a small
portion of the trait variance. This fact leads researchers to focus
on rare-variant mapping with large scale sequencing, which can be
facilitated by using linkage information. The question arises why
linkage analysis often fails to identify genes when analyzing
complex diseases. Using simulations we have investigated the power
of parametric and nonparametric linkage statistics (KC-LOD, NPL,
LOD and MOD scores), to detect the effect of genes responsible for
complex diseases using different pedigree structures. Results As
expected, a small number of pedigrees with less than three affected
individuals has low power to map disease genes with modest effect.
Interestingly, the power decreases when unaffected individuals are
included in the analysis, irrespective of the true mode of
inheritance. Furthermore, we found that the best performing
statistic depends not only on the type of pedigrees but also on the
true mode of inheritance. Conclusions When applied in a sensible
way linkage is an appropriate and robust technique to map genes for
complex disease. Unlike association analysis, linkage analysis is
not hampered by allelic heterogeneity. So, why does linkage
analysis often fail with complex diseases? Evidently, when using an
insufficient number of small pedigrees, one might miss a true
genetic linkage when actually a real effect exists. Furthermore, we
show that the test statistic has an important effect on the power
to detect linkage as well. Therefore, a linkage analysis might fail
if an inadequate test statistic is employed. We provide
recommendations regarding the most favorable test statistics, in
terms of power, for a given mode of inheritance and type of
pedigrees under study, in order to reduce the probability to miss a
true linkage.
Weitere Episoden

In Podcasts werben
Kommentare (0)